Best Practices for Usage-based Billing to Monetize Gen AI
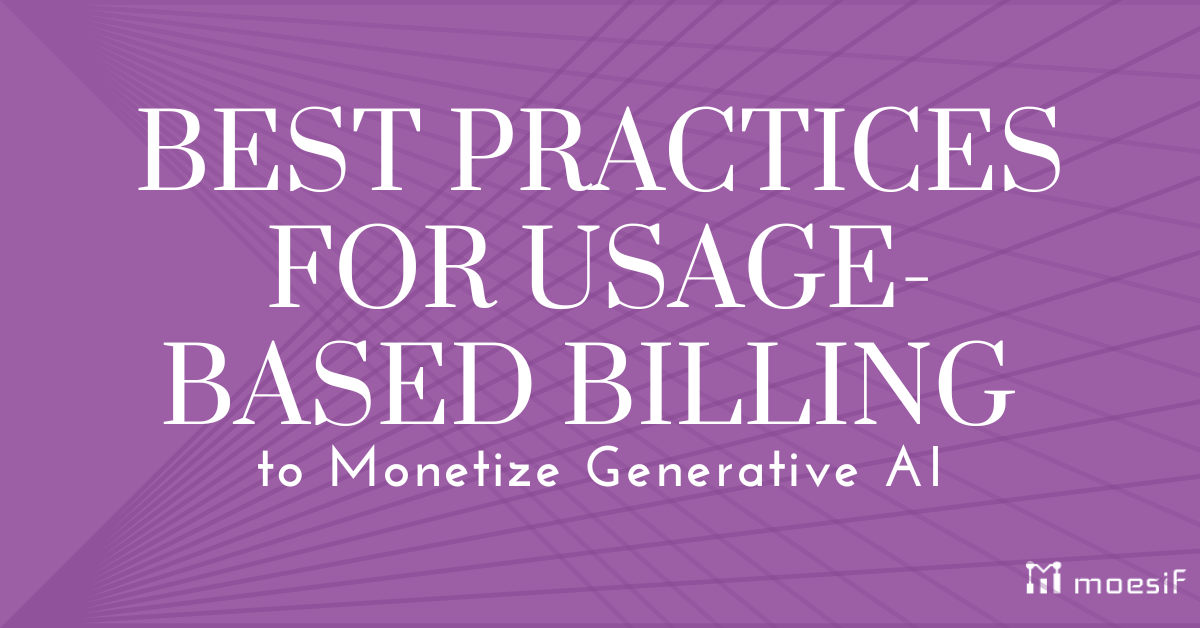
Why monetize APIs?
Artificial intelligence based APIs are reshaping traditional subscription models thanks to their unique monetization frameworks. These API products enable companies seeking tailored solutions in automation and AI workflows, departing from one-size-fits-all UI approaches and embracing a highly customizable experience.
Originally designed for internal platforms, APIs built with AI are now evolving into revenue gateways, transforming them into strategic assets contributing directly to company revenue. These APIs serve as the enablers of integration within diverse systems, fostering interoperability, and enhancing overall operational efficiency. How to make money with AI starts in understanding your AI application’s value in the market.
Repurposing internal functionality to serve external users enhances the value proposition of AI APIs, positioning them as drivers of innovation and profitability in today’s highly competitive SaaS landscape. Monetizing API products can seem like a complex nut to crack, but simply requires the right tools for the right product and a willingness to adapt based on user data.
Challenge of selling AI
So you’re ready to learn how to make money with AI? Selling an artificial intelligence based product is a company-wide endeavor. As organizations shift from traditional on-premises solutions to SaaS and third party solutions, decision-making power moves away from the Chief Information Officer (CIO) to team managers. This trend has only intensified with the transition from SaaS to AI products, empowering individual contributing developers with significant purchasing authority and decision-making power for their machine learning.
While a single developer can research and select an AI product that fits their use case or product need, deploying an app or integration to production involves multiple stakeholder parties. Various teams play a crucial role in the procurement and integration processes for AI products. Even if the intended buyer is not from an engineering department, the technical nature of AI products necessitates communication and input from diverse teams, adding layers of collaboration to the integration process of AI-based APIs.
Land developers first
Pursuing an intricate top-down sales approach to win over every stakeholder is an unnecessary and overly complicated way to foster interest in your AI system. Initiate new business by capturing developers’ interest through a self-serve onboarding experience. By focusing on the intended product users, you can build a more solid case for procurement with developer advocacy.
This developer-first approach doesn’t negate the role of your internal sales team; rather, it focuses on getting developers to recognize the value of an AI powered tool and advocate for it within their leadership team before involving your sales team. Known as developer-first adoption or product-led growth (PLG), the objective is to encourage developers to make a swift and independent decision to test your product, such as $50/month on their credit card. This initial stage doesn’t involve procurement, allowing the subscription to be easily placed on a manager’s credit card. As a result, by the time a developer decides to request a higher seat count or usage limit, your sales team has enough data on the intended company’s use case to build a meaningful Proof of Concept (PoC), strengthening the chance at closing a deal.
Sell through developers
After your customers are actively subscribing to a self-service plan and fulfill specific usage criteria, your sales team can step in. Leveraging the recognized value of your generative AI tool, this sales approach shifts towards consultation, focusing on an “upsell” discussion. After all, the conversation is happening because the potential customer recognized a need for more use of your product. There is less of a focus on “why use our APIs” and more a focus on “leverage our products”, allowing your sales team to guide the customer on optimizing API usage and addressing specific business requirements to explore avenues for increased value. Potential reasons for an upgrade may include heightened usage, the emergence of new use cases, or the presence of advanced requirements that require more API access or different, robust features that are more costly to your own infrastructure.
In the realm of Artificial intelligence, it is crucial for your sales team to be fully aware of a customer’s usage patterns to pinpoint the right moment for engagement in the sales process. To facilitate this, implementation of an API analytics tool is essential. A good API analytics program tracks API usage for each individual customer through an account-based dashboard, offering a comprehensive view of both customer and pilot usage for sales and customer success teams.
Beyond just the analytics side, a good API analytics program will allow you to Implement automatic notifications to offer the best prompt engineering experience. Setting up these alerts through your internal communication platform, such as through Slack, is one advisable way to alert teams of changes in a customer’s usage of your AI powered tool. For instance, a sales discussion might be warranted when an account’s API usage surges by more than 10% week-over-week, signaling a plan with a higher ceiling may be necessary.
Choosing the consumption metric
In the context of AI products, selecting the right metric for usage-based pricing is crucial to align your monetization model with the derived business value for your users. Monetizable AI calls can include those involving predictions, model training, machine learning, data processing, customization, and use of premium features or models. On the other hand, calls for status checks, debugging, test environments, and activities with minimal user value might be less suitable for monetization as there is little incentive for your users to use them. Therefore, billing based on the number of calls sent resulting in a response from your AI technology’s framework proves to be a more effective metric than charging just per API call.
As a secondary example, perhaps you provide a sentiment analysis AI chatbot or AI writing tool running off an internal artificial intelligence framework. You could charge based on the number of text sentiment analysis calls made by users who have purchased your AI integration. But, this could be potentially a bad metric to monetize, especially if these calls are very short or involve minimal machine learning processing. Charging solely based on the quantity of calls might not align with the actual value derived by your API users, as it doesn’t consider the depth or complexity of the sentiment analysis provided or the strain on your internal systems and frameworks. Instead, metrics like the volume of data processed or the level of detail in the analysis can better capture your product’s true value, as it accounts for the complexity of the query/queries in question.
Common value metrics
Name | Example | When to Use | Example Company |
Transaction volume | # of Predictions, # of Recommendations | APIs and recommendation systems | Netflix |
Revenue/cost share | % of Revenue, Transaction Fee | Platforms focused on financial transactions | Stripe |
Data volume | Gigabytes Processed, Records Analyzed | Data-centric platforms such as analytics or processing | IBM Watson |
User-centric | Monthly Active Users | Products charging per user engagement or interaction | |
Resource | Compute Units, Active Hours | Compute infrastructure like cloud services | AWS (Amazon Web Services) |
Ensure the API creates business
Even after successfully onboarding developers to use your AI algorithms, it doesn’t guarantee that your product has inherent business value. Developers might adopt the API for experimental purposes, hobbyist projects, or skill acquisition, and stop there. For AI companies, a basic “Hello World” API is likely not of significance. The primary reason external organizations procure an AI integration service is the perceived value it adds to their current tech stack. In terms of business value, three distinct types are important factors in product acquisition:
- Reduced cost or time (vs a homegrown solution).
- Unlocking of additional revenue for the organization.
- Reduces risk for the organization.
Pricing and packaging
If you’re selling your product, there are many ways to package it depending on your organization’s goals and business ideas. Due to their transactional nature, many APIs thrive under a usage-based billing model (also called Pay As You Go, or consumption-based billing) to kickstart revenue growth. Areas to consider in your pricing strategy include:
- Billing
- Packaging
- Invoicing
1. Billing Strategy
Billing strategy significantly influences adoption and customer activation rates. This planning stage is often guided by product development, particularly in the context of AI. Prepaid billing for an AI platform entails customers paying for a service upfront before utilization, while postpaid billing involves customers settling payment after the services have been consumed.
Prepaid billing
Prepaid billing is a common payment model in the software industry. It involves customers paying for their subscription in advance of utilizing an AI application. In this approach, users commit to a predetermined plan or subscription level (usage limit), making an upfront payment, valid for a specified billing period. This model offers customers cost predictability, as they know the exact amount they will be charged, offering transparency and enabling better financial planning for customers, creating a more content userbase. Software providers, in turn, benefit from improved and consistent cash flow, receiving payments upfront. This process allows businesses to reinvest in their API products and enhance their ability to manage operational expenses efficiently.
Postpaid billing
Postpaid billing offers an alternative payment model, allowing customers to use SaaS services first and pay for them later, typically at the end of a billing cycle. In this arrangement, users are billed based on their actual usage of a product or service during a designated period. Postpaid billing offers flexibility to customers, as they are charged retroactively for the services consumed, making it suitable for those who may prefer assessing their needs before committing to a payment.
However, from the API provider’s perspective, managing cash flow may present challenges, as revenues are collected after services have been rendered. Beyond this, users may be surprised at their actual bill once invoicing is completed, as postpaid billing requires customers to manage their own usage. This means it is advisable to build a dashboard or alerting system of some sort to keep users informed of their usage before a given billing cycle ends. Because you’re extending credit and API use, it can be abused by customers depending on your offering (like a dine and dash scenario). This means it’s critical to have internal usage limits to ensure a customer doesn’t accumulate “too much credit”, before they spend money.
Feature | Postpaid Billing | Prepaid Billing |
---|---|---|
Billing Structure | Fixed charges on a schedule | Upfront payment for usage |
Credit Check | Requires credit check | No credit check |
Flexibility | Less flexibility | More control |
Overage Charges | May incur overage charges | No overage charges |
Contract Commitment | Contract commitment | No contract commitment |
2. Packaging Strategy
In the world of AI, packaging strategy holds significant influence over both the initial contract value and any subsequent expansion of revenue. Given the diverse needs of customers, packaging of AI software can involve presenting various SKUs and offerings that cater to individual requirements based on a PoC. This segmentation may incorporate distinct features or usage components tailored to specific customer needs.
A common packaging technique in the generative AI field is tiered pricing. Akin to SaaS models, organizations offer plans categorized as “good,” “better,” and “best,” each with predefined features and quotas. As price increases, so does feature complexity and quota cap. Another effective approach is the Pay As You Go (PAYG) model, which is really just synonymous with usage-based or consumption-based pricing. In this framework, customers purchase a specific quantity or volume, such as the number of queries made, providing flexibility and serving as a revenue accelerator, particularly for developer-first or product-first organizations.
Tiered pricing
For an AI tool, a traditional tiered pricing model can offer simplicity for customers, enhancing cost understanding and predictability. Widely adopted in the SaaS industry, tiered pricing minimizes billing complexities and requires minimal implementation efforts, often relying on subscription billing software.
However, a notable drawback lies in the potential disparity between price and perceived value. As customers approach plan limits, the necessity to upgrade arises, but the significant price jump to the next tier may lead to hesitancy and a refusal to upgrade. Striking a balance is crucial to prevent “analysis paralysis” and ensure a smooth customer experience. All of this must be weighed against the costs of maintaining your AI model data and frameworks effectively.
Pay As You Go (PAYG) pricing
Pay As You Go (PAYG) pricing offers unparalleled flexibility and cost-efficiency. With PAYG, users pay only for the services they consume, aligning costs directly with actual usage, and therefore with actual value. Additionally, PAYG attracts new customers by lowering entry barriers and encourages exploration without a significant upfront commitment
A benefit of usage-based pricing is that it aligns costs directly with the value derived from a service. Since users pay for the actual consumption of resources or features, there is a guarantee that customers only pay for what they use.
Tiered | Pay As You Go (PAYG) | |
---|---|---|
Description | Traditional SaaS tiers with predefined set of features and quota. | Usage-based or consumption-based pricing based on a unit price. |
Pros | - Enforces a min spend | - Predictable for customer |
- Predictable for customer | - Easy to implement | |
- Easy to implement | - More efficient for customer | |
- More efficient for customer | - Less friction in expansion | |
- Less friction in expansion | - Can “appear” cheaper | |
- Can “appear” cheaper | ||
Cons | - Friction in expansion | - Friction in expansion |
- Rigid, not aligned to value | - Rigid, not aligned to value | |
- Can upset customers with billing surprises | - Can upset customers with billing surprises | |
- Complex to implement | - Complex to implement |
3. Invoicing Strategy
Invoicing strategy plays a crucial role in influencing cash flow and unit economics for an AI tool. The choice between recurring invoicing, with its predictable schedules, and threshold-based invoicing, triggered by predefined limits, shapes how revenue is generated and recognized. You can also invoice customers once customers reach a threshold such as when they reach a certain quota or outstanding spend. This type of invoicing is called threshold-based invoicing.
Recurring invoicing
Recurring invoicing involves a systematic approach in which customers are billed on a regular schedule. This predictable billing model provides customers with a clear understanding of their financial commitment and the time constraints on their API usage. From a business perspective, recurring invoicing contributes heavily to stable cash flow and simplifies financial forecasting thanks to guaranteed revenue on a guaranteed timeline. While this model is widely adopted in the world of SaaS and is appreciated for its predictability, it can pose challenges in scenarios where extreme usage patterns lead to low-cost transactions.
Threshold-based invoicing
Threshold invoicing operates on a different paradigm compared to recurring invoicing. In this monetization framework, invoices are triggered only after a customer surpasses a predefined threshold, such as a specified volume of usage. This approach offers flexibility, allowing customers to pay for services only when they have reached a certain level of consumption. However, threshold invoicing can introduce complexities in accounting and revenue recognition. The time frame between invoicing events becomes less predictable, making it challenging for financial planning and positioning your AI product as a negative opportunity that could potentially result in diminished revenue compared to the potential earnings achievable with a recurring model.
Recurring | Threshold | |
---|---|---|
Description | Customer invoiced on a schedule like each month, quarter, or year | Customer invoiced only after a credit threshold is met (can be prepaid also) |
Pros | - Easier for revenue recognition/GAAP | - More predictable |
- More predictable | - Reduced transaction cost | |
- Reduced transaction cost | - Makes prepaid easier | |
- Makes prepaid easier | ||
Cons | - Bad unit economics for low-cost SaaS | - Complex if prepaid |
- Complex if prepaid | - Harder for finance to recognize revenue | |
- Harder for finance to recognize revenue | - Company liability |
Implementing usage-based pricing
Implementing usage-based pricing for an AI tool requires planning and adaptability. Establishing a robust tracking mechanism, integrating analytics tools, and automating notifications are key steps to success. Finding a value metric closely tied to your user’s needs can ensure that the invoicing of your API product aligns with the perceived value for your users, fostering fairness and trust within your customers. Success lies in creating a user-centric experience that reflects your product’s value based on your user data and analytics.
You can build your own data warehousing on a platform like Snowflake or you can use a purpose-built usage-based billing product for APIs like Moesif. With an external solution, connect to your API gateway of choice like Kong or Tyk and then integrate it with a billing provider like Stripe to easily set up metering rules for the best AI monetization solution. For AI startups who need to invest directly in their product, outsourcing analytics is a natural fit for AI technology. For a detailed tutorial on how to do this with Kong and Stripe, check out the End-to-end API Monetization with Kong, Stripe, and Moesif.